Summary
This detailed guide will introduce you to AI analytics, highlight how it diverges from traditional analytics methods, and explore the critical elements and advantages it provides. You will also learn about the evolution of reporting through AI analytics and its potential impact across different sectors and job functions, including its notable benefits for call centers.
Upon completing this guide, you will gain a comprehensive understanding of AI analytics' role in enhancing business performance and elevating customer satisfaction levels.
Understanding AI Analytics
AI analytics revolutionizes how we understand large datasets, utilizing machine learning to discern patterns, trends, and crucial relationships without human intervention. This innovative approach empowers companies to make informed, data-driven decisions, staying ahead in the competitive market.
Machine learning, a pivotal element of AI analytics, is a subset of artificial intelligence that enables computers to learn from data independently, without being explicitly programmed. It employs sophisticated algorithms and models to uncover patterns and predict outcomes, enriching the data analysis process.
This field of analytics is divided into several categories, including supervised, unsupervised, and reinforcement learning, each offering unique applications and presenting distinct challenges.
Equally important in AI analytics is natural language processing (NLP), the technology that allows computers to interpret and generate human language. NLP sharpens AI analytics by analyzing unstructured data–like text, speech, and images–to draw valuable insights. This technology includes sentiment analysis, which can identify the motions expressed by speakers.
NLP further enhances the user experience through natural language search capabilities, enabling queries in everyday language and delivering precise answers from vast datasets.
Beyond analysis, AI analytics employs data visualization techniques to present findings in a straightforward and captivating manner. Effective data visualization aids in revealing patterns and anomalies, facilitating easier understanding and sharing of information. Through interactive features such as filters, sliders, and various charts, users can tailor the data presentation to their specific needs.
🔥Ringover Tip
Sentiment analysis is an AI feature provided by Empower by Ringover which identifies emotions expressed by speakers, so your team won’t miss any signals sent during interactions.
What's the Difference Between AI Analytics and Traditional Analytics?
AI analytics and traditional analytics serve the common purpose of analyzing data to inform decision-making. Their differences lie in areas such as automation level, data types, analytical techniques, and the nature of questions they address.
AI analytics stands out for its high level of automation and velocity, capable of digesting large data volumes promptly. This facilitates real-time or near real-time insights, devoid of human intervention, thus empowering businesses with the agility for data-driven decision-making to stay ahead in the competitive landscape. Conversely, traditional analytics tends towards slower data processing, primarily manual, posing the risk of missed opportunities due to delayed insights.
When it comes to data types, AI analytics boasts the ability to manage both structured and unstructured data formats, including text, speech, and images, extracting valuable insights. Additionally, it efficiently analyzes streaming and real-time data from sources like sensors, social media, and web logs. Traditional analytics, however, is limited to structured data and often struggles with unstructured formats. It necessitates that data be pre-stored in databases or data warehouses for analysis.
The methodology also differs significantly between the two. AI analytics employs sophisticated algorithms and machine learning models for pattern identification and prediction, utilizing methods such as clustering, anomaly detection, natural language processing, and data visualization for enhanced data exploration and presentation. Traditional analytics, however, relies on structured data and conventional statistical methods, like regression and correlation analysis, suitable for historical data interpretation and addressing descriptive and diagnostic inquiry.
Furthermore, the scope of questions each can address varies; AI analytics is adept at tackling predictive and prescriptive queries, offering forecasts and personalized recommendations based on data and user preferences. Traditional analytics confines itself to answering "what happened?" and "why did it happen?", focusing on factual information and historical interpretation without forecasting or suggesting actions.
Key elements of AI analytics
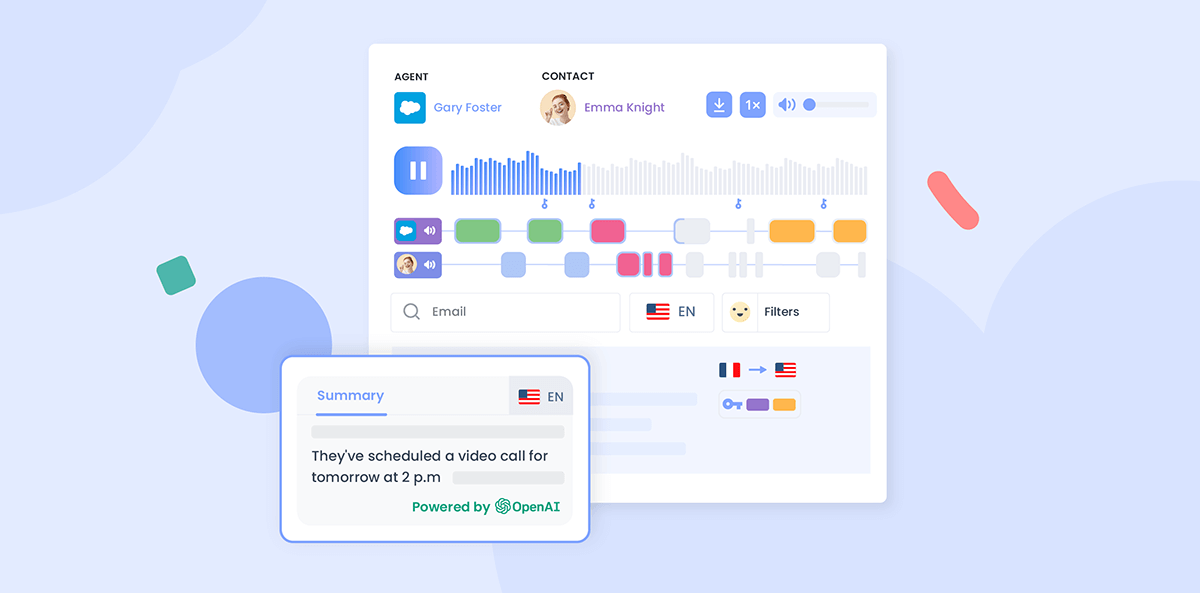
AI analytics encompasses a series of critical components, ranging from the origins of data and its preparation to sophisticated analysis and the extraction of insights. Below, we delve into each of these integral elements, shedding light on their role in the efficacy of AI analytics.
Data sources are the cornerstone of AI analytics, constituting the various origins of data collection. These sources can be characterized as internal or external, structured or unstructured, as well as static or dynamic. They encompass a wide range of platforms, including but not limited to databases, data warehouses, data lakes, APIs, web pages, social media platforms, sensors, and more. The diversity, quality, and volume of these data sources directly influence the outcomes of AI analytics, impacting the accuracy and dependability of the results.
The process of data preparation and collection stands as the next pillar in AI analytics. This encompasses the aggregation, purification, and amalgamation of data from disparate sources. The challenges in this phase are manifold, particularly when confronted with vast datasets, a variety of formats, and issues related to data quality. AI analytics facilitates this process through the employment of algorithms designed to assess, validate, and refine data. These algorithms propose techniques for data enhancement, such as the elimination of duplicates, anomalies, or missing entries.
Data analysis represents the third pillar, where AI techniques and algorithms are applied to scrutinize and interpret data, drawing insights, and forming predictions or recommendations. This process leverages various AI disciplines, including machine learning, natural language processing, data mining, and data visualization.
- Machine learning equips computers with the capability to learn from data autonomously, without explicit programming.
- Natural language processing enables computers to comprehend and generate human language.
- Data mining involves identifying patterns and correlations within large data sets.
- Data visualization facilitates the representation of data in visual or interactive formats.
The culmination of AI analytics lies in the insights and decisions derived from data analysis. These insights can address a spectrum of queries, including descriptive (what happened), diagnostic (why something happened), predictive (what will happen), and prescriptive (what actions should be taken). AI analytics specializes in furnishing specific, pertinent, and actionable insights, ultimately aiding organizations in augmenting their operational efficiency and customer satisfaction.
Evolution of AI Reporting
Reporting is a vital process that involves sharing information and insights with stakeholders including investors, regulators, customers, and employees. Its purposes vary from informing and persuading to complying with rules. Over time, reporting has transitioned into diverse formats like analytics dashboards, financial statements, annual reports, press releases, and presentations.
The transformation in reporting has been propelled by advancements in technology, analytics, and changing expectations. Initially, reporting was a manual, static process, predominantly based on past events and paper-based documents. It aimed at delivering factual data and adherence to established standards, albeit often suffering from delays, inconsistencies, and errors.
In contrast, modern reporting has embraced automation, becoming more dynamic and forward-looking. It utilizes cutting-edge technologies like artificial intelligence (AI), cloud computing, and data analytics to enhance data processing and presentation. The goal is to provide actionable insights that facilitate decision-making, characterized by improved timeliness, accuracy, and reliability.
AI, in particular, has transformed reporting through:
- Generating content: AI employs natural language generation (NLG) to produce coherent text and images from data, crafting financial reports, press releases, and articles based on diverse data sources and predefined templates. Also, it can generate visual content such as charts and infographics to elucidate key points.
- Analyzing data: Machine learning (ML) and data mining applied by AI enable the analysis of intricate data sets to discover patterns, trends, and anomalies. Functions like sentiment analysis, anomaly detection, and predictive analytics provide insights into data from various sources, including social media and sensors.
- Enhancing quality: Through natural language processing (NLP) and data validation, AI improves the reliability and quality of content by checking for errors in spelling, grammar, and tone. It also verifies data accuracy, completeness, and detects any fraudulent activities.
The contribution of AI analytics in call centers
AI analytics significantly boosts call center software capabilities in enhancing customer service, agent performance, and operational efficiency. Through the application of advanced conversation intelligence software, which uses natural language processing, speech recognition, and machine learning, call centers can streamline and improve numerous tasks, including call center coaching, transcription, call center sentiment analysis, and more.
A key player in this field is Empower by Ringover, a cutting-edge conversation intelligence tool. Empower by Ringover, seamlessly integrating with Ringover and other business applications, furnishes detailed transcripts and summaries of calls. It adeptly identifies the key topics discussed and uses statistics and recommendations to elevate conversation quality.
Empower by Ringover assists call centers in several ways:
- Enhancing customer satisfaction: By analyzing sentiments and emotions expressed by both customers and agents, Empower by Ringover offers actionable advice to enhance communication dynamics. It detects signs of both satisfaction and dissatisfaction, covering compliments, complaints, or requests, and provides real-time feedback and alerts to both agents and their supervisors.
- Boosting agent productivity: Empower by Ringover streamlines post-call workflows like summarizing calls, updating CRM databases, and organizing follow-up actions. It also supplies key call performance metrics—such as talk time, periods of silence, interruptions, and keywords usage—supporting agents in honing their communication skills and efficiency.
- Scaling operations: Designed to manage vast call volumes and datasets, Empower by Ringover delivers immediate insights and reports. Its capability to integrate with a wide array of tools and platforms, including ATS like Bullhorn, Vincere, Recruit CRM, and more, facilitates effortless synchronization and collaboration.
Conclusion
AI analytics equips you to efficiently process and analyze vast data sets, extract meaningful insights, and pursue data-driven decision-making. Moreover, it enhances customer service, agent productivity, and overall operational effectiveness.
To fully leverage the capabilities of AI analytics in revolutionizing your business, consider embracing Empower by Ringover. This conversational intelligence tool seamlessly integrates with Ringover, among other business platforms, offering insightful call transcripts and summaries, immediate topic identification, and enriched conversation quality through detailed analytics and actionable recommendations.
Embark on transforming your business operations with a free trial of Empower by Ringover today, and firsthand experience the difference it can make in achieving your aspirations.
AI Reporting FAQ
What is AI in analytics?
AI in analytics refers to applying artificial intelligence techniques and algorithms to automate the analysis of data, uncover insights, and generate predictions or recommendations. This innovation can significantly reduce costs, enhance the precision of analyses, and improve the quality of decisions for businesses.
What does an AI analyst do?
An AI analyst specializes in leveraging artificial intelligence and machine learning to sift through and interpret complex digital datasets. These professionals support better decision making and boost operational efficiency through sophisticated analytics and statistical techniques. They are also tasked with transforming data-driven insights into strategic plans that fuel business growth and spur innovation.
What AI can analyze data?
AI utilizes a wide array of algorithms and methods to analyze, understand, and draw conclusions from extensive and intricate datasets. Prominent AI tools employed in data analytics include ChatGPT, Empower by Ringover, Tableau, Power BI, Microsoft Excel, Jupyter AI, Polymer, MonkeyLearn, Qlik Sense, and Kanaries RATH.
What is the difference between data analytics and AI?
The primary distinction between data analytics and AI lies in their focus and capabilities: data analytics aims to analyze historical data to discern insights and guide decisions. In contrast, AI extends into the realm of assumption, learning, and prediction, offering insights and recommendations that surpass human capability. While data analytics provides a descriptive analysis based on past occurrences, AI is prescriptive, suggesting optimal future actions.